Assistant Professor
Department of Mathematics
University of California, San Diego
9500 Gilman Drive #0112
La Jolla, CA 92093
Office: APM 5755
Email: xctian at ucsd.edu
Google Scholar page
Curriculum Vitae (Sep 2023)
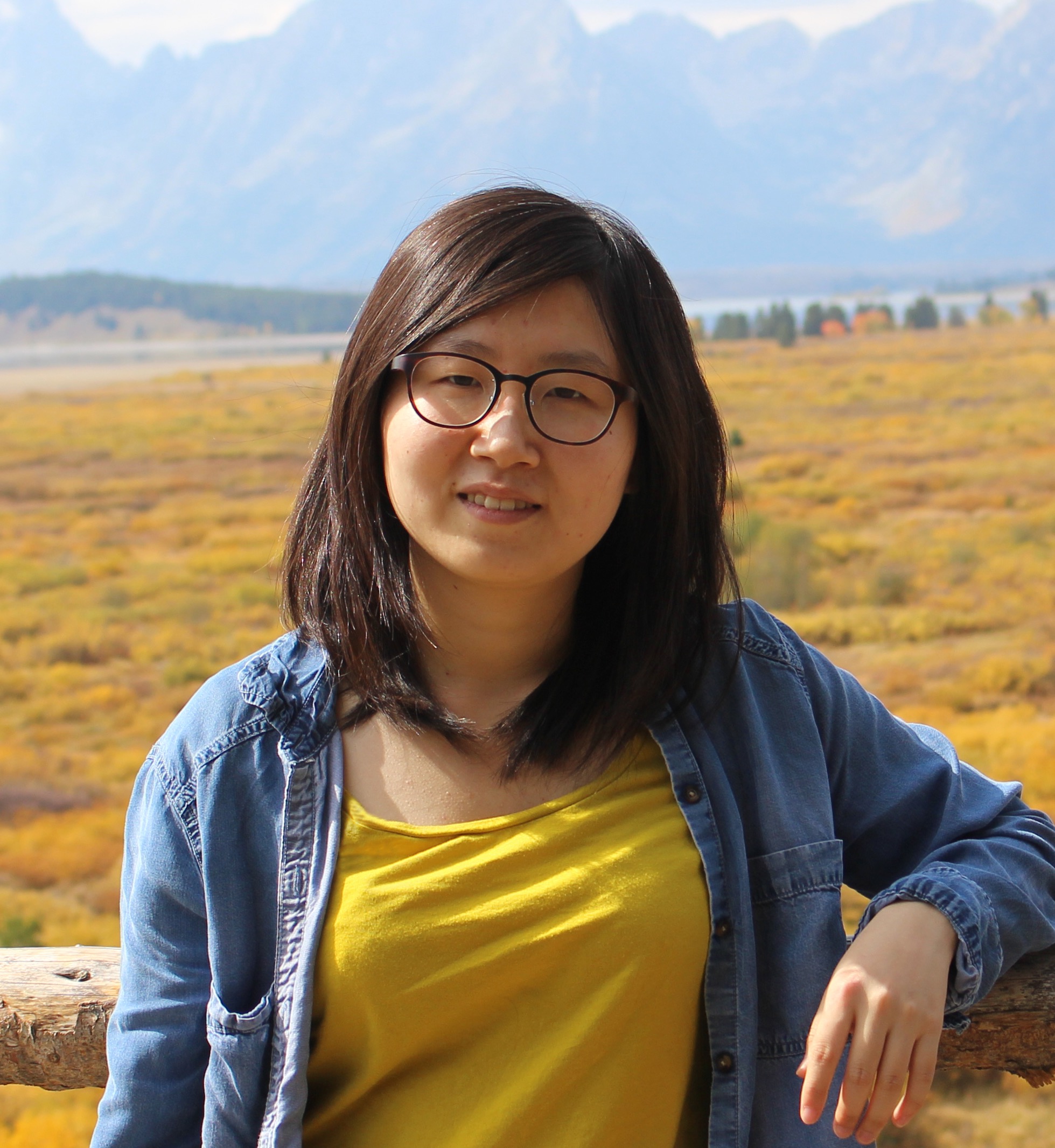